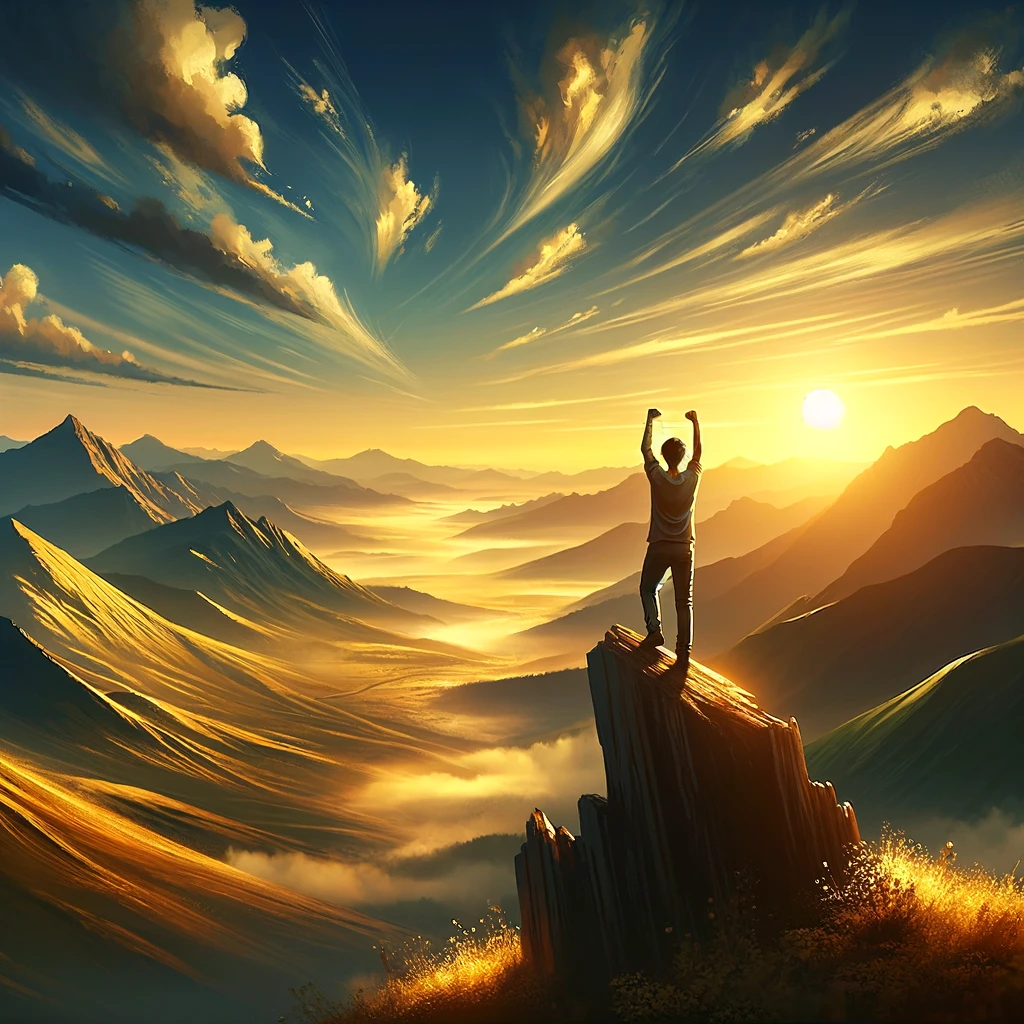
Essential Steps to Become the Best Version of Yourself
SEO Meta Description
Discover the transformative journey to becoming the best version of yourself with practical steps on self-improvement, emotional intelligence, and lifelong learning. Start your path to personal growth today.
Understanding Personal Growth
The journey to becoming the best version of yourself is both exhilarating and challenging. It involves diving deep into your inner world, understanding your desires, and overcoming the fears that hold you back. It’s about pushing boundaries, learning continuously, and embracing change.
Self-Assessment: The Foundation of Growth
Self-assessment is your first step. By identifying your strengths and acknowledging your weaknesses, you set the stage for meaningful improvement. It’s about being honest with yourself and setting clear, achievable goals that guide your path forward.
Mindset Mastery: Cultivating a Growth Mindset
Adopting a growth mindset is pivotal. It means seeing challenges as opportunities, failures as lessons, and constantly seeking ways to better yourself. This mindset fosters resilience, encouraging you to persevere through setbacks and keep striving for your goals.
Physical Well-being: A Pillar of Personal Development
Your physical health is a cornerstone of personal development. Regular exercise, balanced nutrition, and adequate rest not only improve your body’s function but also enhance your mental clarity and emotional stability. It’s about respecting your body and giving it the care it deserves.
Emotional Intelligence: Navigating Life with Awareness
Developing emotional intelligence is crucial. It enables you to understand and manage your emotions, build stronger relationships, and navigate the complexities of social interactions with empathy and insight. It’s about being in tune with yourself and those around you.
Skill Development: Lifelong Learning
The pursuit of knowledge and skills is never-ending. Whether it’s enhancing your current abilities or learning something entirely new, continuous education keeps you relevant and adaptable. It’s about embracing the journey of learning as a lifelong adventure.
Time Management: Maximizing Productivity
Effective time management transforms how you work and live. By prioritizing tasks, setting realistic deadlines, and eliminating distractions, you can achieve more with less stress. It’s about making the most of your time to focus on what truly matters.
Financial Literacy: Securing Your Future
Understanding finances is key to securing your future. From budgeting and saving to investing wisely, financial literacy empowers you to make informed decisions, ensuring a stable and prosperous life. It’s about taking control of your financial destiny.
Social Connections: The Role of Relationships
Building and maintaining healthy relationships are vital to personal growth. These connections provide support, offer new perspectives, and open doors to opportunities. It’s about nurturing bonds that enrich your life and the lives of others.
Mental Health: The Core of Personal Fulfillment
Taking care of your mental health is non-negotiable. Whether it’s managing stress, seeking professional help when needed, or practicing mindfulness, prioritizing your mental well-being is essential for a balanced and fulfilling life. It’s about valuing your inner peace as much as your external achievements.
Spirituality and Inner Peace
Finding spirituality or a sense of inner peace is a deeply personal journey. It can provide a grounding force, offer comfort during tough times, and help you understand your place in the world. It’s about connecting with something greater than yourself, whatever that may be for you.
Creativity and Innovation: Unleashing Potential
Creativity isn’t just for artists; it’s a crucial skill for problem-solving and innovation. Encouraging creative thinking in all areas of your life can lead to unexpected solutions and new possibilities. It’s about seeing the world through a lens of curiosity and openness.
Leadership and Influence: Impacting Others
Developing leadership skills isn’t just for those in managerial positions. It’s about influencing others positively, whether in your personal life, community, or workplace. Good leadership is ethical, empathetic, and transformational. It’s about being the change you wish to see.
Habits for Success: Building a Better You
Your daily habits form the foundation of your life. Cultivating positive habits and shedding negative ones can significantly impact your well-being and success. It’s about making small, consistent changes that add up to big transformations.
Adventure and Experience: Growing Beyond Comfort Zones
Stepping out of your comfort zone is where growth happens. Whether it’s traveling to a new country, trying a new activity, or simply changing your routine, new experiences challenge you and expand your perspective. It’s about embracing the unknown with open arms.
Contribution and Community Service
Giving back to your community is both rewarding and enriching. Volunteering your time or resources can make a significant difference in the lives of others and provide a sense of fulfillment and connection.
FAQs
How do I start my journey to personal growth? Begin with self-reflection to understand your current position and where you want to be. Set small, achievable goals to start moving in the right direction.
Can personal growth happen at any age? Absolutely. Personal growth is a lifelong process that knows no age limits. It’s never too late to start.
How do I measure my progress? Set clear, measurable goals and regularly review them. Celebrate your successes, and learn from your setbacks.
What do I do if I feel stuck? Seek new experiences, learn new skills, or consider working with a mentor or coach. Change your routine to spark creativity and find new inspiration.
How important is a support system? A strong support system is invaluable. Surround yourself with people who encourage and uplift you.
How can I maintain motivation? Keep your goals visible, celebrate progress, and remember why you started. Find a community or group with similar aims for mutual support.
Conclusion
Becoming the best version of yourself is a journey filled with challenges, learning, and growth. It requires dedication, patience, and resilience but rewards you with a fulfilling life rich in experiences, relationships, and achievements. Remember, personal growth is not a destination but a continuous process of becoming who you wish to be. Embrace each step with openness and optimism, and never stop striving for improvement.